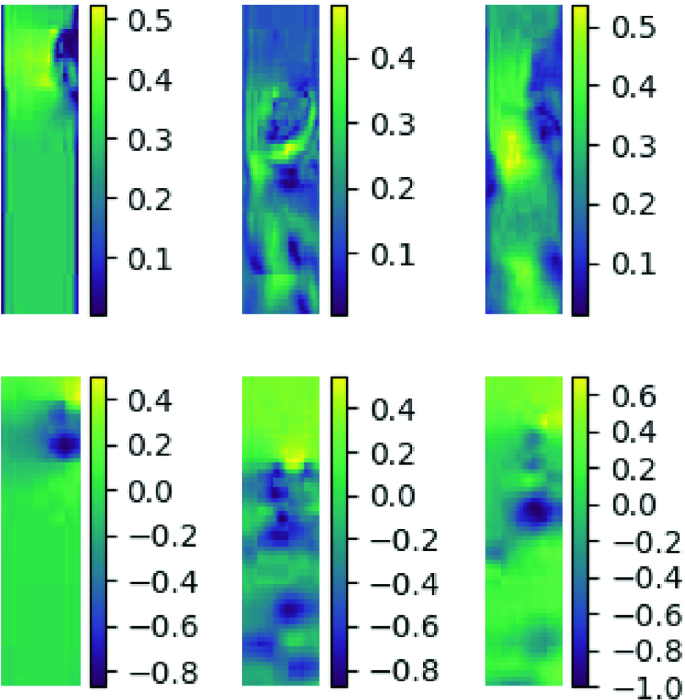
Deep Convolutional Generative Adversarial Networks Applied to 2D Incompressible and Unsteady Fluid Flows | SpringerLink

Deep Fluids: A Generative Network for Parameterized Fluid Simulations - Kim - 2019 - Computer Graphics Forum - Wiley Online Library
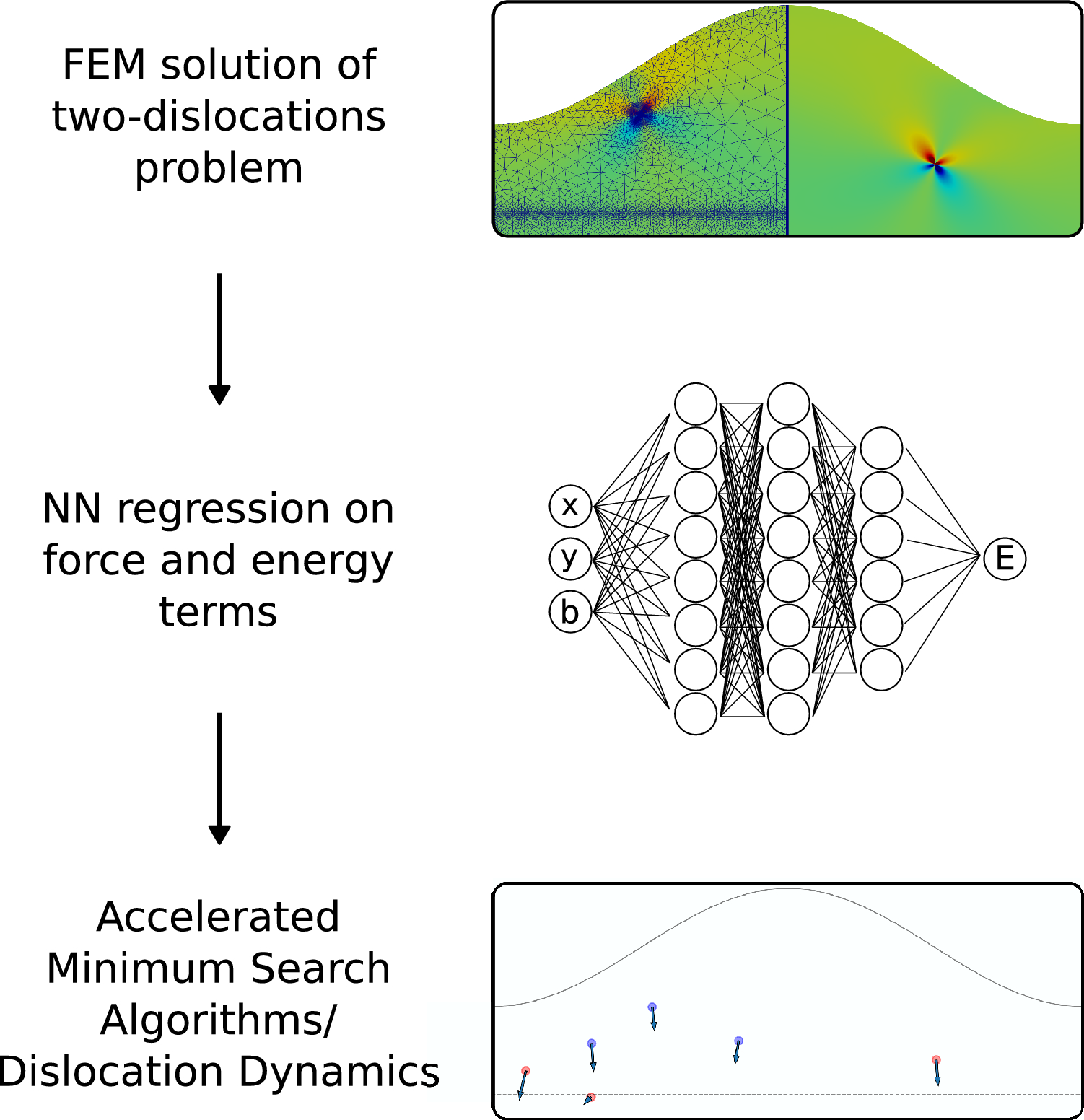
Machine learning potential for interacting dislocations in the presence of free surfaces | Scientific Reports
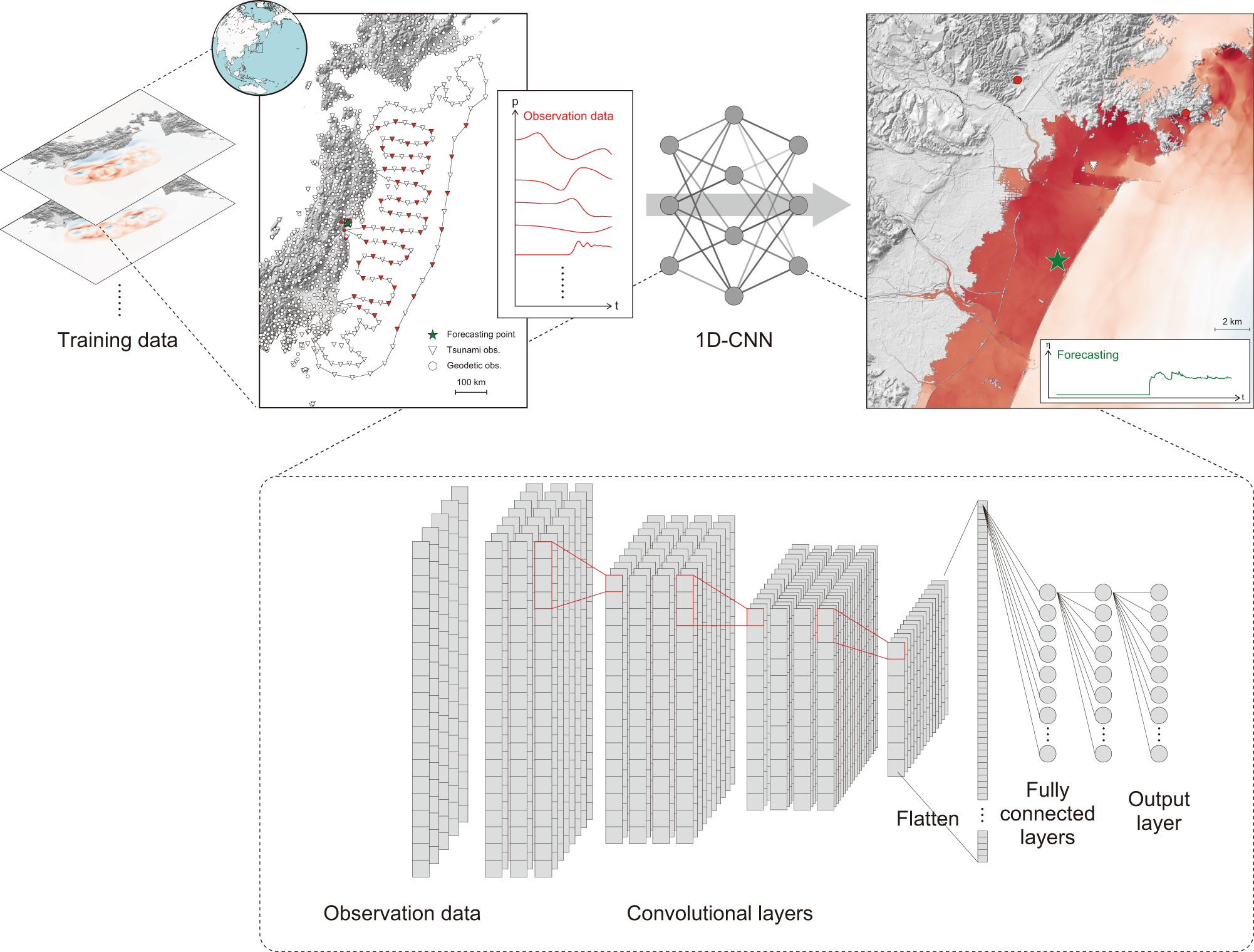
Early forecasting of tsunami inundation from tsunami and geodetic observation data with convolutional neural networks | Nature Communications

Shallow neural networks for fluid flow reconstruction with limited sensors | Proceedings of the Royal Society A: Mathematical, Physical and Engineering Sciences

An advanced hybrid deep adversarial autoencoder for parameterized nonlinear fluid flow modelling - ScienceDirect
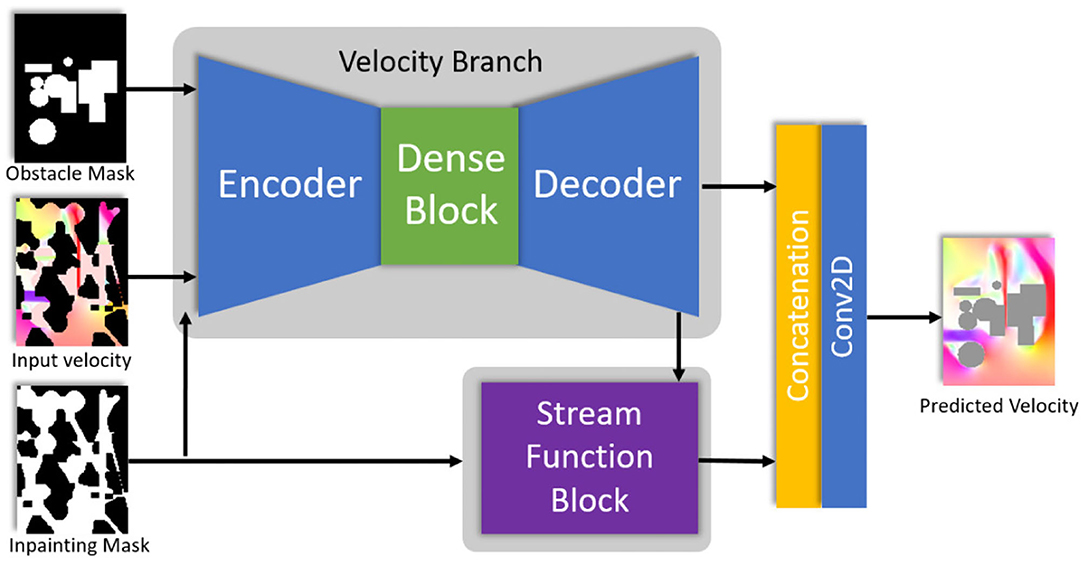
Frontiers | A Physics-Aware Neural Network Approach for Flow Data Reconstruction From Satellite Observations | Climate

Teaching the incompressible Navier–Stokes equations to fast neural surrogate models in three dimensions: Physics of Fluids: Vol 33, No 4
